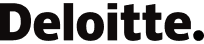
Predictive Market Intelligence
Predictive Market Intelligence
Deloitte Case study
100k
100k
Data points daily
Data points daily
3x
3x
More possible deal flow
More possible deal flow




Project Overview
Industry
M&A Consulting
AI Solution
Co-Occurrence LLM
Quick Brief
Deloitte’s Emerging Technology and Global Markets team was looking to detect M&A activity ahead of the market. They were on the look out for ways to detect and gain access to the latest corporate M&A in real time.
Customer Pain Points
Reliant on public new reports
No edge over competition
Slow reaction times
What We Delivered
2 AI modules that took data from company profiles and news sources to see if companies were increasingly linked. We then used AI reasoning to give M&A alerts to relevant cases.
End Results
Edge over competition
Enhanced decision making
Opened new possible strategies
Introduction
Developing a competitive edge in M&A deal-flow is something that very few companies have been able to do effectively. Banks and corporate advisors are constantly on the look out for ways to detect and gain access to the latest corporate M&A in real time.
Using market signals and company reports as prediction forecasters is another painstaking challenge, but having an early indication on merger activity can be highly valuable to anyone whose business relies on this sort of market activity.
The Solution
We set out to measure the co-occurrence of company entities in public news in combination with a module that analyses up-to-date company strategy, resulting in a score that is able to indicate possible future M&A activity.
The Perlon solution required considerable data collection, cleaning and reprocessing to allow for the Models and Functions to create actionable data. NLP components including LLMs, embedding, and Named Entity Recognition (NER) models were all used to achieve the final output.
The core components consist of:
Strategy Module
Initial indication of suitability based on known strategic direction of selected companies. Uses company profiles through an embedding model and an LLM to create a pairwise M&A score and an M&A distance score.
Co-occurrence Module
NER used on company profiles + scraped & preprocessed news data. Entity and contextual vector embedding models + TF-IDF are then used to create a co-occurrence score.
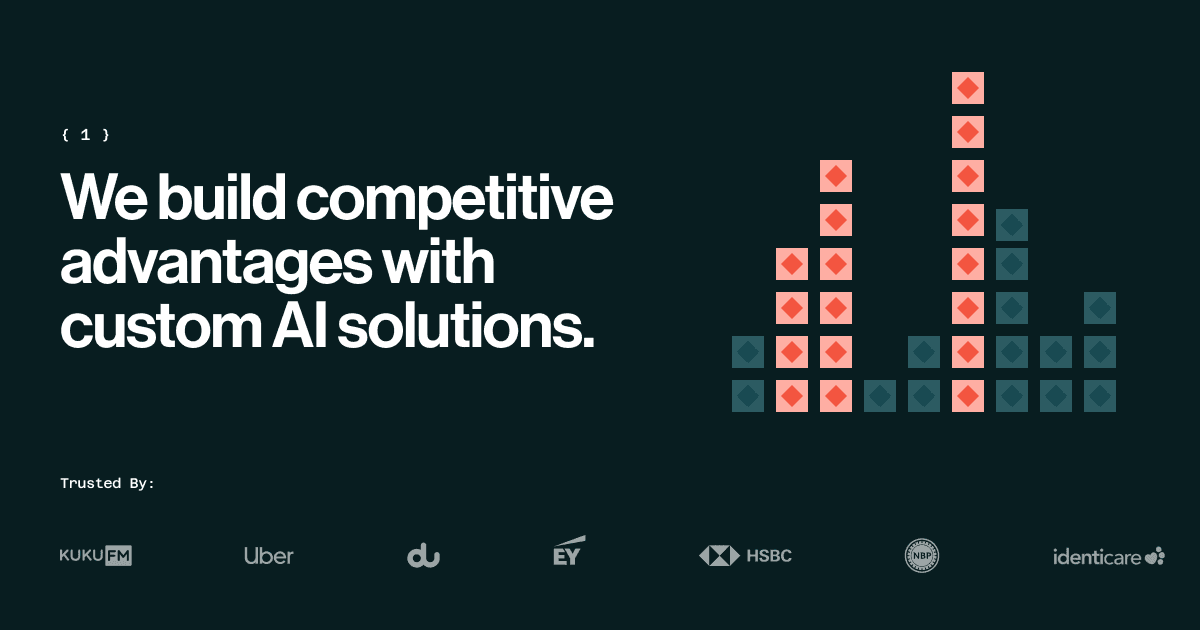
Result
A highly intuitive system that is able to provide insights and market signal which would otherwise be impossible to detect.
Project Overview
Industry
M&A Consulting
AI Solution
Co-Occurrence LLM
Quick Brief
Deloitte’s Emerging Technology and Global Markets team was looking to detect M&A activity ahead of the market. They were on the look out for ways to detect and gain access to the latest corporate M&A in real time.
Customer Pain Points
Reliant on public new reports
No edge over competition
Slow reaction times
What We Delivered
2 AI modules that took data from company profiles and news sources to see if companies were increasingly linked. We then used AI reasoning to give M&A alerts to relevant cases.
End Results
Edge over competition
Enhanced decision making
Opened new possible strategies
Introduction
Developing a competitive edge in M&A deal-flow is something that very few companies have been able to do effectively. Banks and corporate advisors are constantly on the look out for ways to detect and gain access to the latest corporate M&A in real time.
Using market signals and company reports as prediction forecasters is another painstaking challenge, but having an early indication on merger activity can be highly valuable to anyone whose business relies on this sort of market activity.
The Solution
We set out to measure the co-occurrence of company entities in public news in combination with a module that analyses up-to-date company strategy, resulting in a score that is able to indicate possible future M&A activity.
The Perlon solution required considerable data collection, cleaning and reprocessing to allow for the Models and Functions to create actionable data. NLP components including LLMs, embedding, and Named Entity Recognition (NER) models were all used to achieve the final output.
The core components consist of:
Strategy Module
Initial indication of suitability based on known strategic direction of selected companies. Uses company profiles through an embedding model and an LLM to create a pairwise M&A score and an M&A distance score.
Co-occurrence Module
NER used on company profiles + scraped & preprocessed news data. Entity and contextual vector embedding models + TF-IDF are then used to create a co-occurrence score.
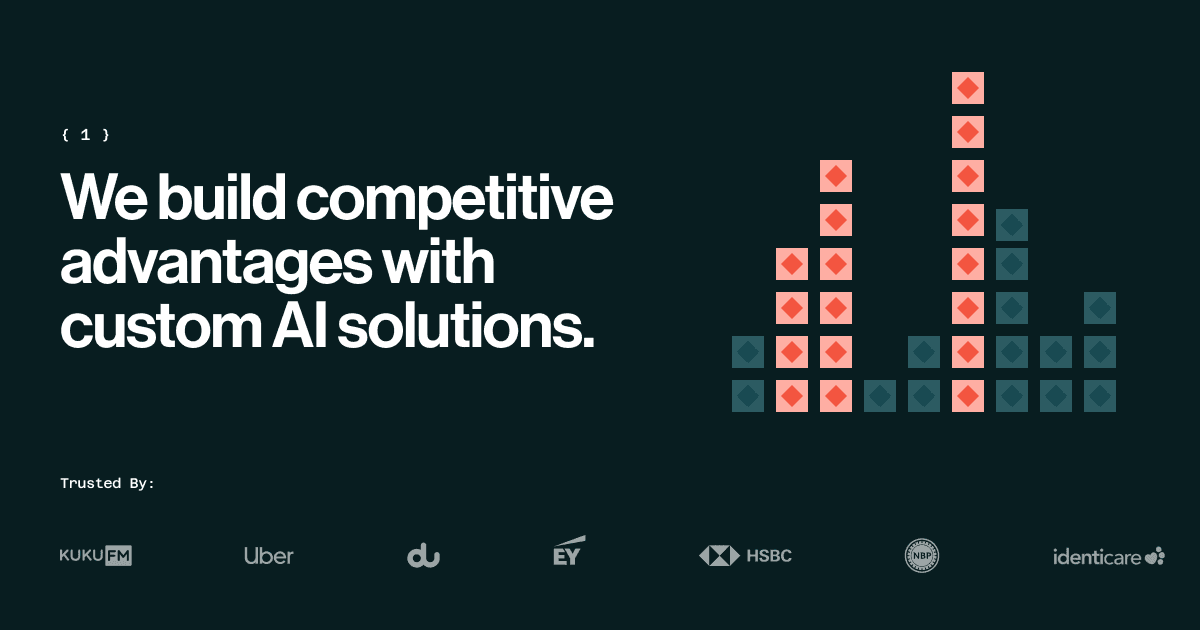
Result
A highly intuitive system that is able to provide insights and market signal which would otherwise be impossible to detect.
More case studies
More case studies

Du Telecoms
Customer Service Analytics
Customer Service Analytics
Industry
Telecoms
AI Solution
Customer Service Analytics
Quick Brief
Du were looking to acheive full visibility on the operations of their call centres and retail stores by analysing the ongoing performance of customer service teams in both Arabic and English.
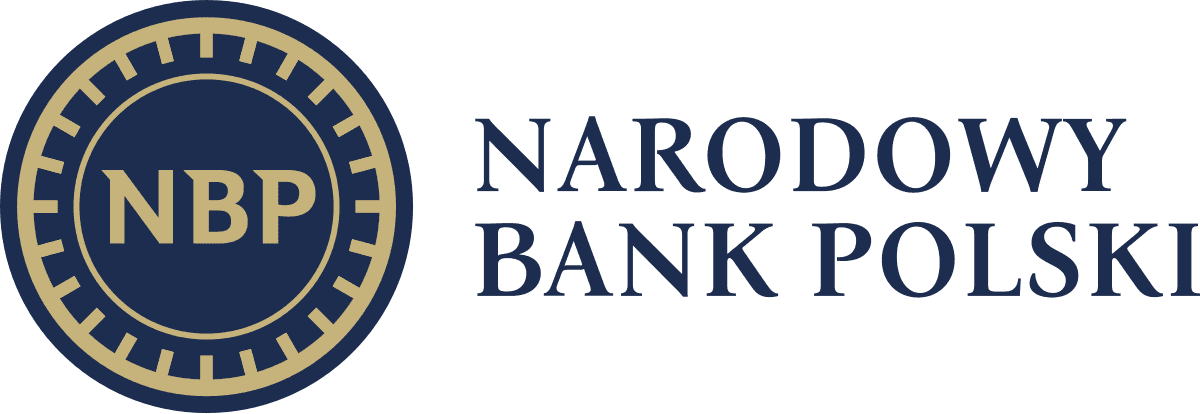
Bank of Poland
Document Translation
Document Translation
Industry
Banking
AI Solution
Multi-Language Document Translation
Quick Brief
NBP wanted a secure way of translating customer documents in 8 langauges through a centralised employee platform.
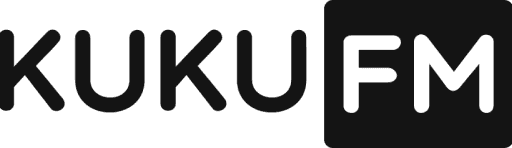
KukuFM
AI Audiobook Generation
AI Audiobook Generation
Industry
Media
AI Solution
Generative Text-to-Speech
Quick Brief
KuKuFM wanted to reduce the cost of using human voice artists and increase the speed of bringing new audio content to market.

Jets.com
Custom AI for Outbound Sales
Custom AI for Outbound Sales
Industry
Private Aviation
AI Solution
Outbound propsecting LLM
Quick Brief
Develop an AI platform that is able to send prospects hyper-personalised sales and marketing emails at scale.
© 2025 Perlon AI Ltd (DBA as Perlon Labs). All rights reserved.
© 2025 Perlon AI Ltd (DBA as Perlon Labs). All rights reserved.
© 2025 Perlon AI Ltd (DBA as Perlon Labs). All rights reserved.
© 2025 Perlon AI Ltd (DBA as Perlon Labs). All rights reserved.
© 2025 Perlon AI Ltd (DBA as Perlon Labs). All rights reserved.